Synthetic data: The key to maintaining AI supremacy while upholding individual rights
Synthetic data not only mimics real-world data, but it also does not contain sensitive personal information. And, this is no longer a theoretical concept
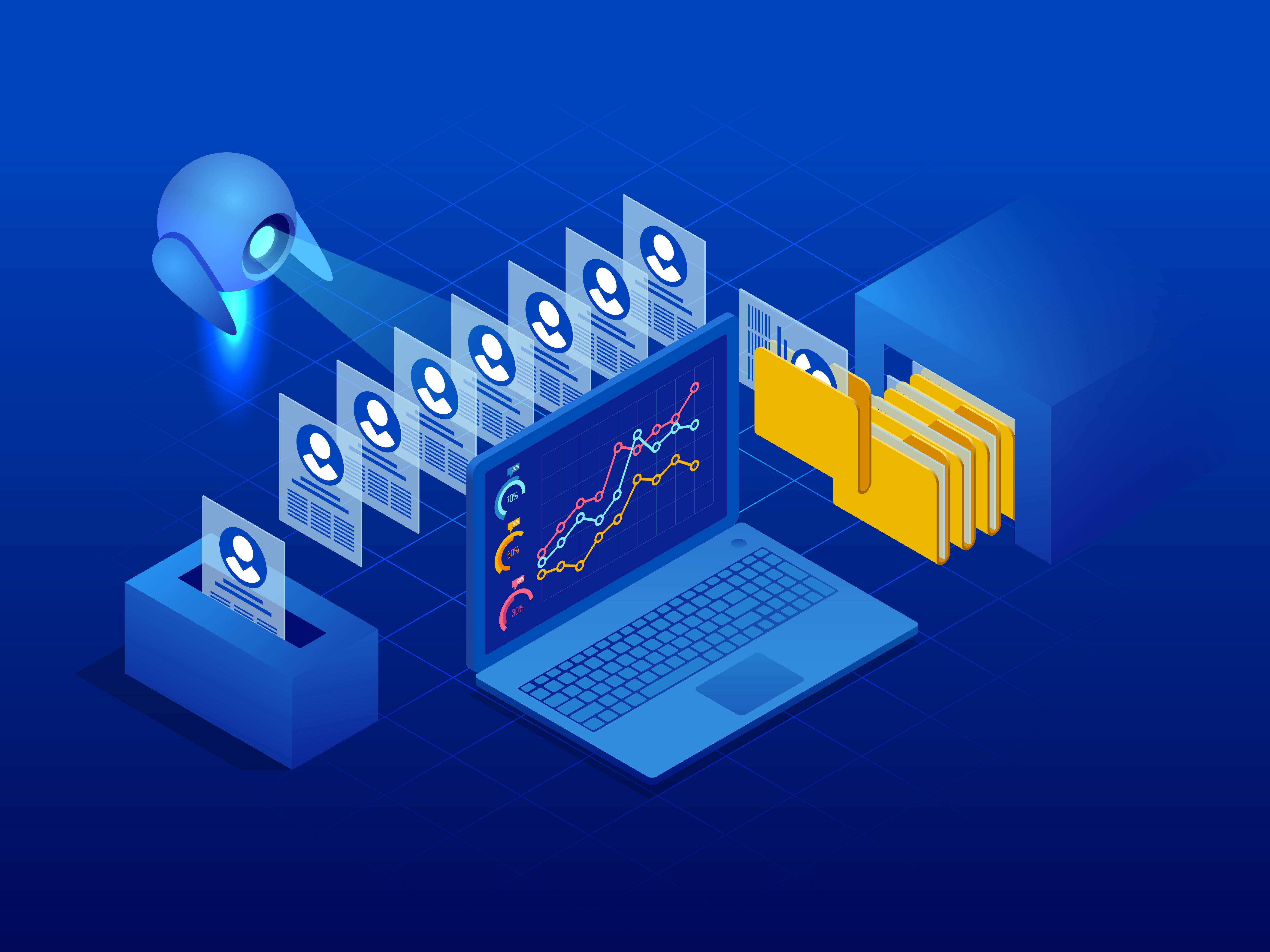
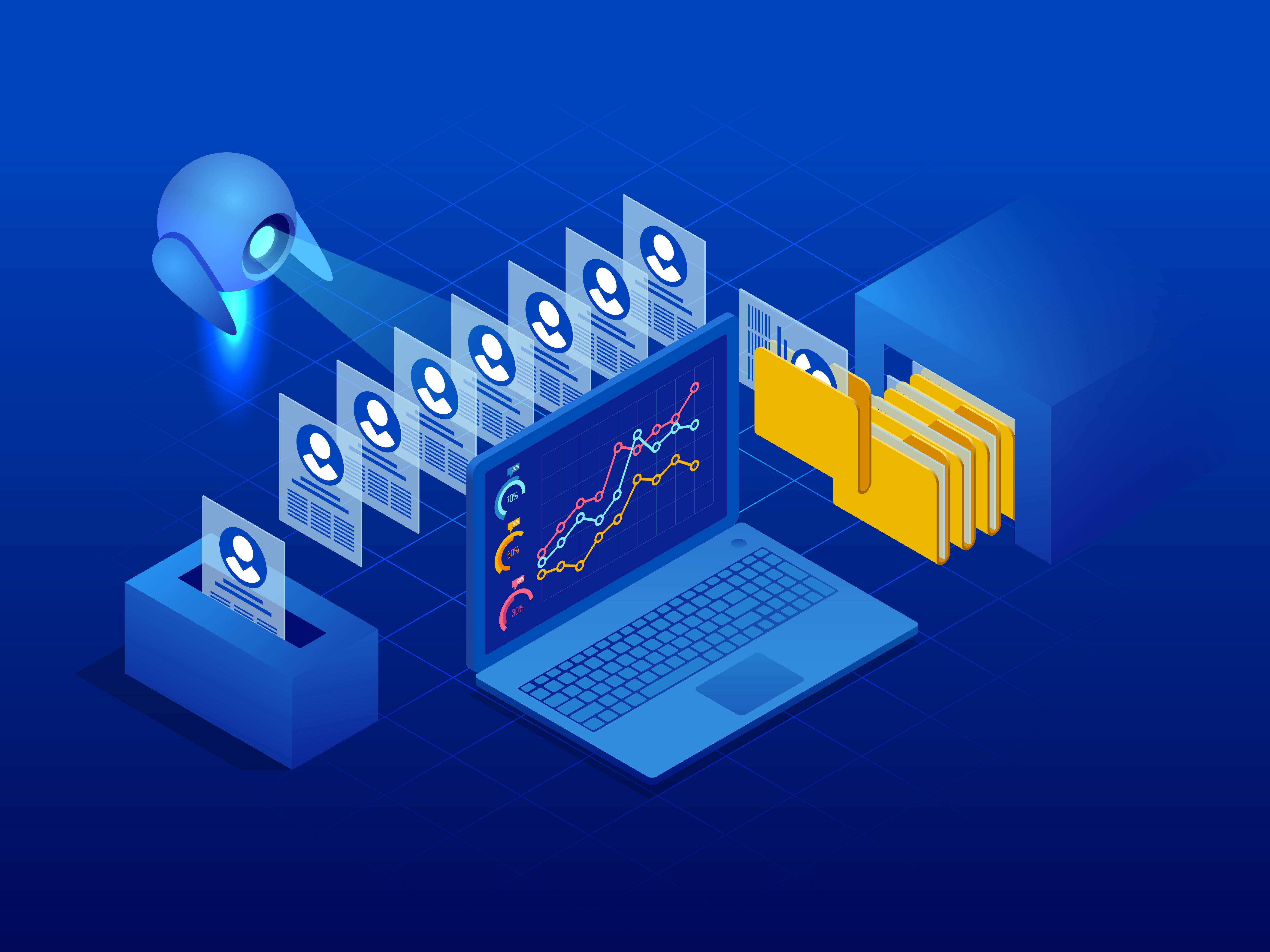
The race for AI supremacy is not only a technology contest but a race for geopolitical and economic supremacy. With various estimates indicating a growth of $7 trillion over 10 years by Goldman Sachs, or $2.6-$4.4 trillion annual growth by McKinsey’s, or predicting that AI will affect 40% of all jobs, as suggested by the IMF—every nation is doing its best to gain AI leadership.
In this high-stakes race for dominance in AI, Western nations must compete while upholding the flags of individual rights, which are enshrined in strict regulatory frameworks like GDPR in Europe or CCPA in California. Is this a choice between democratic values and technological progress?
The answer lies not in abandoning our principles but in embracing innovation. Artificially generated and well-labelled, synthetic data is the answer to real data. Synthetic data not only mimics real-world data, but it also does not contain sensitive personal information. And, this is no longer a theoretical concept.
The power and viability of synthetic data were compellingly showcased in June 2024 when NVIDIA unveiled its Nemotron-4 340B Instruct model. Remarkably, this cutting-edge model was trained using a dataset comprised of 98% synthetic data, yet it achieved performance metrics on par with, and in some cases exceeding, those of models trained extensively on real-world information.
This breakthrough signals a paradigm shift: synthetic data is not just a stopgap but potentially a superior, more efficient route to high-performing AI.
AI models have been used for long by hedge funds and institutions for a long time. China’s DeepSeek R1 AI model was supposedly trained on GPUs acquired by its parent entity, which was a hedge fund.
In banking and finance, client data cannot be shared with AI models as the models can be reverse-engineered to extract personally identifiable financial data. This limits training AI models to detect financial fraud or uncover connections between entities. We cannot use AI models to detect transactions triggered by malevolent entities unless we are able to train AI models with enough examples. Finance is not the only industry where it is difficult to get real data.
The healthcare and life sciences industry has more stringent regulations regarding data privacy than finance. While AI models can help us unravel genetic structures, discover new drugs, and find patterns among thousands of patient data points, their potential is hardly utilised owing to the lack of real patient data.
With the help of synthetic data, this shortcoming can be largely overcome. It is not only the privacy laws that warrant the use of synthetic data, but also the paucity of data on rare disease patients.
AI models cannot be trained on rare diseases if they haven’t encountered enough relevant data points. Western democracies can still excel at cutting-edge medical research through AI without giving up patient data, if they only embrace synthetic data.
Of course, critics may argue that synthetic data can never fully replicate the nuanced richness of real-world information and what the models will learn will never be the reality. While this concern holds a degree of validity, it perhaps misses the larger strategic picture.
Astronauts train for spacewalks in underwater environments that simulate zero gravity; these simulations are not space, but they are exceptionally effective for learning critical tasks. Similarly, AI systems can learn essential patterns and relationships from meticulously constructed synthetic environments, achieving high performance levels without compromising an individual's privacy.
The growing consensus in the tech industry on using synthetic data is evident in Microsoft’s Azure Foundry and Amazon’s AWS Bedrock, which offer ways to create synthetic data that can be used for training AI models. No wonder, research firm Gartner has predicted that by 2030, a staggering 40% of all data used for AI training will be synthetic.
For Western democracies, especially the United States, synthetic data is not just a clever technical fix. It is a pathway to sustain AI technological leadership in a manner which is consistent with democratic principles. The future of AI must be intelligent and ethical; synthetic data is the key to unlocking that future.
To quote an old Chinese proverb—It doesn’t matter if the cat is black or white, as long as it catches the mice.
Pawan Prabhat and Paramdeep Singh are the Co-founders of Shorthills AI
Edited by Suman Singh
(Disclaimer: The views and opinions expressed in this article are those of the author and do not necessarily reflect the views of YourStory.)